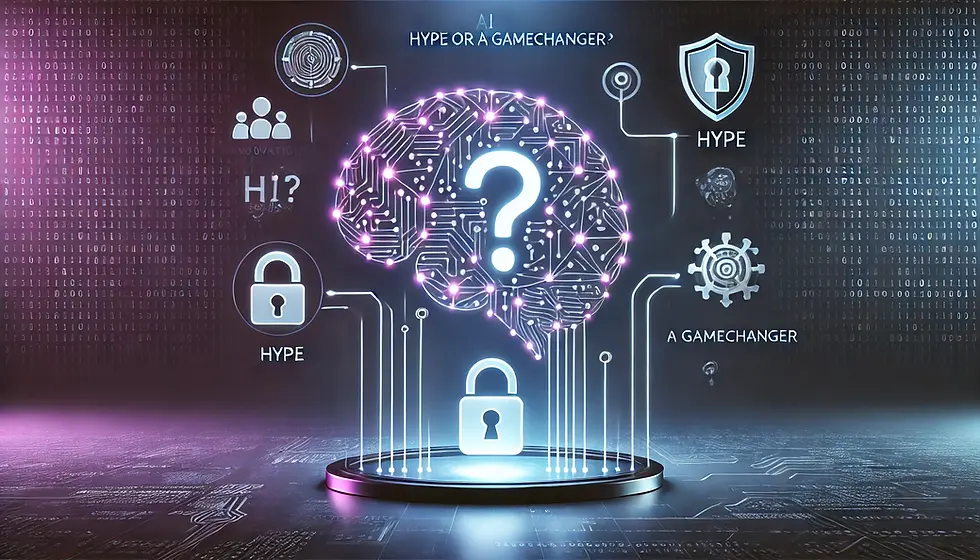
Image generated by OpenAI's DALL·E
Can artificial intelligence (AI) really make the difference, or is it just another buzzword that offers more marketing than substance? In an industry that is also characterized by staff shortages, the use of AI is one of the most exciting and controversial approaches. Companies are investing millions in AI-based security solutions, but the question remains: is the benefit really worth the effort and resources?
The threat situation is changing rapidly and becoming increasingly complex. Classic approaches are increasingly reaching their limits, and this is precisely where AI promises to help: It should be able to detect anomalies, analyze threats and respond to attacks in a fraction of a second.
But how often can these promises actually be kept in practice – and where does the benefit end when reality overtakes expectations?
Do we really need AI in cyber security?
The integration of artificial intelligence into cyber security is polarizing: while some see it as an indispensable tool for tackling modern challenges, others consider it an overrated buzzword that offers more marketing than benefit. To answer this question, it is worth taking a look at the possible uses - and limitations - of AI in practice.
The following focuses on four key advantages of AI in cyber security and just as many critical counterarguments that highlight the limitations and potential risks of this technology.
Where AI makes sense...
Detection of anomalies
AI models have the ability to analyze large amounts of data and identify behavioral patterns that could indicate attacks. AI shows its strengths especially when it comes to unknown threats such as zero-day attacks.
Example: Detecting unusual data transmissions on a particular network segment that indicate a possible attack.
Automated Threat Analysis
Given the huge amount of security data generated every day, AI helps separate important alerts from irrelevant ones, counteracting "alert fatigue" among security teams.
Example: A company invests in an "AI-powered" vulnerability management solution that offers little more than standard metrics such as CVSS scores in a new design. The added value remains low, while the AI label unnecessarily increases costs.
Phishing and malware detection
AI can identify patterns in emails or files that indicate phishing attempts or malicious payloads. This is particularly valuable in attacks that aim to bypass human protections.
Example: Automated filtering of emails that appear deceptively genuine but contain malicious links or attachments.
Personalized defense measures
By analyzing user and device behavior, AI can customize protective measures and thus ward off attacks in a targeted manner.
Example: An AI system recognizes that a certain server suddenly records high levels of access to sensitive data outside of normal business hours. Instead of blocking access completely, the action is marked for review and additional approval is requested from an administrator.
Where AI is questionable...
Marketing-driven AI solutions
Many products are labeled "AI-powered" but are based only on rule-based systems or simple algorithms. The focus is often more on sales strategy than on real technological innovation.
Example: A security tool is marketed as "AI-powered" but is based only on static rules and heuristics. The AI label is used to justify a higher price even though no real AI features are implemented or relevant.
Overengineering
In many cases, proven methods such as rules or signatures can detect threats just as effectively - and at a much lower cost. AI models do not offer any significant improvement here, but often only increase complexity. AI should not be used as an end in itself. In many cases, it is better to use simple and robust solutions.
Example: A classic firewall log analyzer often delivers sufficiently accurate results without the need for an AI system.
Ethics and transparency
Many AI systems are "black boxes" whose decision-making processes are incomprehensible. In an area where accountability and accountability are essential, this represents a significant weakness.
Example: An AI system for threat detection was trained with incomplete data that incorrectly classifies certain user groups, ways of working or countries as riskier. This could lead to legitimate activities being blocked or certain people being disproportionately alerted.
Data security and data protection
AI requires large amounts of training and real-time data. This poses risks in terms of privacy and data security, especially when sensitive information is processed.
Example: An AI system that analyzes network traffic could inadvertently detect sensitive information such as credit card details or passwords and store them in log files, which then become unauthorizedly accessible in the event of a data leak.
A recent example that highlights the risks of AI-based systems is the Microsoft Copilot incident, where permission issues led to employees gaining access to their managers' emails. This case shows that even advanced AI systems can make mistakes that pose significant data protection risks.
Conclusion
AI in cyber security is neither a panacea nor a superfluous buzzword - its role lies somewhere in between. Used correctly, AI offers significant advantages such as the detection of anomalies, automated threat analysis and support in prioritizing measures. But at the same time, it entails risks such as a lack of transparency, high costs and the danger that marketing promises outweigh the actual benefits.
We should therefore not view AI as an end in itself, but rather use it as a targeted tool in a comprehensive security strategy. The key is to set realistic expectations, identify the right use cases and see AI as a complement to human expertise rather than a replacement. This is the only way it can really unfold its added value.